SAMPLING OF COPPER : “AN ECONOMIC CHALLENGE FROM ROCK TO CATHODE”
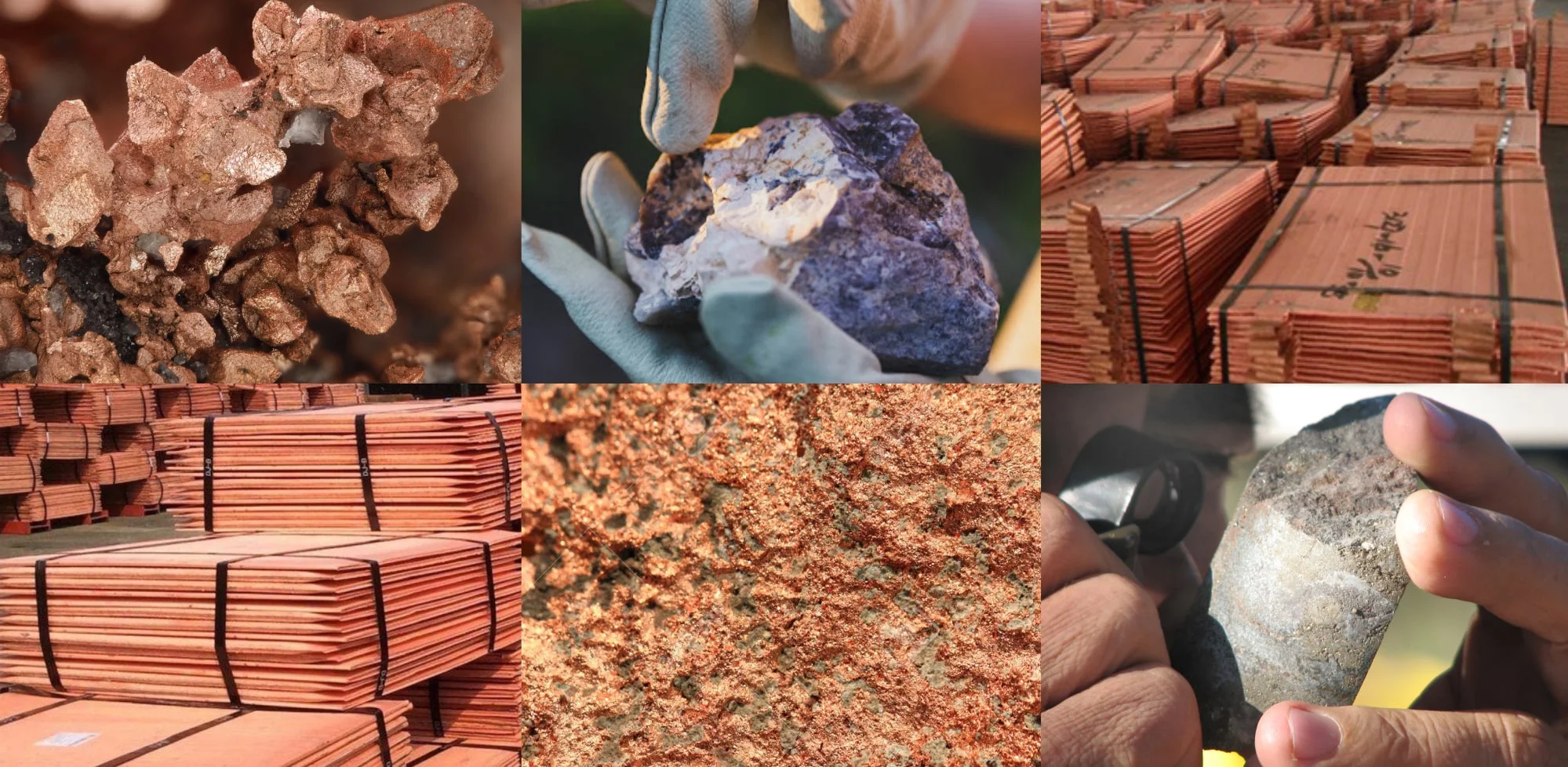
INTRODUCTION
Major discrepancies between mine estimates and estimates from plant metallurgical accounting are a common problem in many copper operations. Furthermore, discrepancies between estimates from the mine plants and smelters can also be severe. Many existing practices are based on practical protocols that often fall short of basic statistical requirements. Sampling stations and tools are often flawed by design and cannot provide the needed reliability for achieving accurate ore grade control, ore reserves, and believable metallurgical accounting. These problems are amplified by the naive application of correcting factors.
An 18-point strategy is provided in this course to minimize these reconciliation problems. The strategy is based on accurate sampling capable of providing reliable data, on thorough statistical evaluations identifying the causes of variability, random or non-random, and on a Total Quality Management philosophy offering a platform for proactive decisions.
Executive-supported strategies founded on integrated Sampling, Statistical Process Control, Geostatistics, and Total Quality Management, and the discipline to enforce them is an unambiguous directive to engineers and operators. Invariably, this progressive and knowledgeable attitude from the executive team provides the leadership that will uncover many previously hidden problems creating devastating and invisible financial losses, all along the way from rocks to cathodes.
COURSE CONTENT
Day One
Point #1: Create a plan to capitalize on existing data. Existing chronological data are free and a gold mine of information. Yet, more often than not, nobody looks at them in depth for the possibilities they offer to top management.
Lecture 1: An introduction to variography (a convenient tool for metallurgists).
Point #2: Use ISO standards as a structural framework for implementing preventive maintenance. However, ISO standards are not standards for good correct Sampling, Statistical Process Control, Geostatistics, and Total Quality Management. Iso standards are only logical organizational guidelines.
Lecture 2: Introduction to the 9 kinds of sampling errors.
Point #3: Do not misuse ISO standards. Symptoms of misuse are:
- Status quo is the rule.
- Employees lose their creativity.
- The certification agency runs the company.
- Employees refer to ISO standards to protect and justify their actions, or lack of them.
- Management pursues only the ISO certification.
- The biggest achievement of ISO standards results in too much emphasis on metallurgical accounting.
Lecture 3: Do a new lecture.
Point #4: Integrate good Sampling, Statistical Process Control, Geostatistics, and Total Quality Management into a single program under the responsibility of a manager of standard mining processes.
Point #5: Implement a philosophy of Equiprobabilistic Sampling. When this philosophy is not endorsed by top management, many sampling biases with their devastating negative effects necessarily take place, resulting in confusion, loss of time, and heavy financial losses. In this lecture four real cases are presented where financial losses due to bad sampling were quantified.
Day Two
Point #6: Do not confuse quantity of work with real productivity. As far as correct sampling is concerned, geologists, metallurgists and chemists must learn how to do the job right the first time.
Point #7: Establish realistic Data Quality Objectives for every process controlling parameter. Logical Data Quality Objectives cannot be improvised, they are the result of a careful variability analysis. Presentation of a useful guideline to help in real practice.
Point #8: Work smarter, not harder. A good understanding of the various kinds of heterogeneity and the variability they generate in the process helps to implement a successful Statistical Process Control program.
Point #9: Beware of economic units within the same company. Management learned that independent economic units perform better as they become more responsible for a cost-effective performance. But it is not without danger, as all these units work in a logical, inescapable chronology, where the performance of one greatly affects the performance of the next one. Some economic units may learn to perform better by exporting their problems to the next unit.
Point #10: Establish a continuous feedback system with customers. Customer and process feedback (e.g., feedback between miners and metallurgists) must be the object of careful statistical analyses using chronological data plots, moving averages, variograms and their derived functions, and variographic control charts. Presentation of real cases.
Day Three
Point #11: Make a distinction between a non-random process fluctuation and a random sampling/measurement fluctuation.
Point #12: Constantly minimize invisible cost.
Point #13: Beware of correcting factors. The problem is that there is no such thing as a constant sampling bias. Correcting factors only give a false sense of security to management.
Point #14: Implement a creative thinking cycle.
Point #15: A mine/mill reconciliation problem nearly always has multiple cause:
- Geological model causes.
- Mining causes.
- Ore grade control causes: Explanation why precision alone can lead to devastating financial losses.
- Mill and flotation plant causes: Real cases where non-probabilistic sampling systems were installed.
- Laboratory causes: A real case where XRF assays for copper led to a devastating lawsuit.
Day Four
Point #16: A good understanding of variability must improve metal recovery. Every mine which had a management endorsed ore blending policy observed great improvement at the mill and flotation plant within one year
Point #17: Use pragmatic statistical improvement tools based on graphic observation. Complex statistical analyses often fall short of transmitting a clear message to the users at the mine and the mill
Point #18: Use concrete graphic tools to quantify invisible cost. Many people still believe that a reconciliation problem between the mine and the mill must be the result of an accuracy problem generating a bias. They are wrong; precision alone can generate a massive reconciliation problem, therefore massive financial losses
Conclusion: All these critically important points will be discussed using appropriate lectures, and give valuable knowledge to the course participants.
Dr. FRANCIS PITARD
Dr. Francis F. Pitard is a consulting expert in Sampling, Statistical Process Control and Total Quality Management. He is President of Francis Pitard Sampling Consultants (www.fpscsampling.com) and Technical Director of Mineral Stats Inc. (www.mineralstats.com) in Broomfield, Colorado USA. He provides consulting services in many countries. Dr. Pitard has six years of experience with the French Atomic Energy Commission and fifteen years with Amax Extractive R&D. He taught Sampling Theory, SPC, and TQM for the Continuing Education offices of the Colorado School of Mines, the Australian Mineral Foundation, for the Mining Department of the University of Chile, and the University of Witwatersrand in South Africa. He has a Doctorate of Technology from Aalborg University in Denmark. He is the recipient of the prestigious Pierre Gy’s Gold Medal for excellence in promoting and teaching the Theory of Sampling (Cape Town, South Africa, 2009). Consultant of InterMet for Peru. Chairman of II Mineral Sampling Congress to be held in Lima on September 2021.